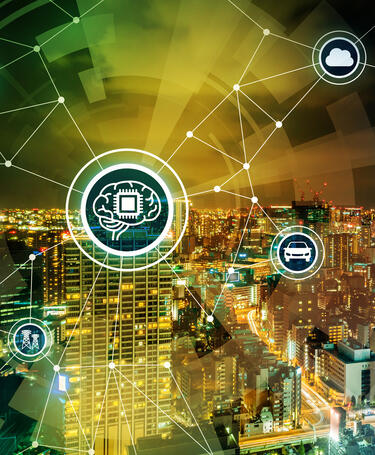
Using machine learning to make a business case for quality
Progress indicator
The application of artificial intelligence may sound like a complex concept reserved for tech giants, but embracing it can benefit the quality function.
Machine learning is the application of artificial intelligence (AI) whereby computer systems are capable of picking up on algorithms without being explicitly programmed. It is a field of computer science that enables systems to “learn”.
“When we think about AI and data analytics the first thing that comes to mind is Cambridge Analytica, Facebook and Google adverts – the sinister edge,” said Richard Corderoy, CQP MCQI, and Partner at Oakland Consulting. “What seems to get lost is how we can apply these tools to make a business case for quality to the board.”
According to Corderoy, there are two branches of machine learning: supervised and unsupervised. In supervised learning, computer systems are given a data set of work examples. In manufacturing, it can be used to predict whether batches are good or bad. Most of the data that quality professionals use with their clients falls under this branch. In unsupervised learning, the system clusters and profiles users based on their behaviour. The way tech giants such as Amazon function fall under this category; for example, the adverts displayed to users are based on their interests as suggested by their online clicking patterns.
“The power of data and the way we thought the world works is changing,” said Corderoy. “There is a better way than Excel spreadsheets that allows us to make a more compelling [business case] to the management team.”
The power of data and the way we thought the world works is changing.
Today’s technological advances have resulted in the intense proliferation of data. According to the World Economic Forum, 90% of all data has been created in the last two years, and the world produces 2.5 quintillion bytes per day. This vast amount begs the consideration of what data is actually valuable. According to Corderoy, there are six dimensions involved in considering the quality of data across an organisation’s value chain:
- Timeliness: Is the available data the most up-to-date?
- Validity: Were the proper measurements taken in gathering data?
- Consistency: Do duplicates exist?
- Integrity: Are relations between entities and attributes coherent?
- Completeness: Was all the necessary data collected?
- Accuracy: Does the data come from verifiable sources?
“Data analysis [provides] ammunition to talk the language of the business,” said Robert Oakland, CQP MCQI, and Partners at Oakland Consulting. “[Quality professionals] play an absolutely key role in coaching, mentoring and [driving] fact-based decision-making. It helps us understand the costs of our quality efforts.”
New ways of looking at old-school problems
A major limitation of relying on traditional methods for data analysis, such as Excel, is that a lot of relevant data could be excluded and difficult to verify.
Corderoy encourages taking the following four-step approach to analytics:
- Think through the problem: Define the issue and spend time to think through it thoroughly.
- Check the data exists: Understand what data you need to solve the issue.
- Do the analysis: Use the right tool(s) for the job.
- Implement outcomes: Know how you are going to use the output.
He emphasised the importance of asking clear questions by asking yourself “How would I coin this problem so I could give it to a child to solve? Can you predict in this sample how many will pass or fail?”
Power BI, Qlik, Tableau Software, PredictSis and Microsoft Azure are among business intelligence software that can identify areas that will impact business the most, such as how failure can be reduced, or how to improve yield. For example, Microsoft Azure is a cloud-computing service that quality professionals can use as a problem-solving tool to identify root causes and factors. The platform can identify missing values, compare algorithms, create a boosted decision tree, capture false positives and determine which data set has the most impact on business.
“This is the holy grail of statistical process control,” Corderoy said, adding it can measure huge areas of operational performance, production in factories, the service in services firms, and spot customers about to leave based on their behaviour, and identify key talent.
Quality World
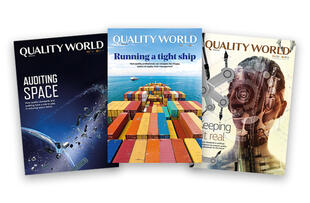
Get the latest news, interviews and features on quality in our industry leading magazine.