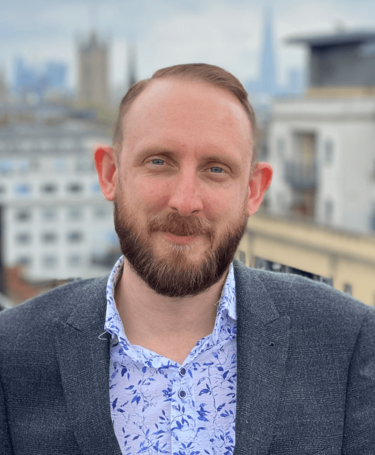
Challenges of ensuring quality in AI
Progress indicator
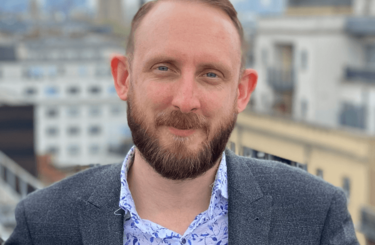
With artificial intelligence now an established part of our working lives, Damien Tiller explains how quality professionals can ensure quality is baked in when AI processes are implemented.
As a quality professional, it is impossible not to have encountered some form of artificial intelligence (AI) or machine learning (ML), whether in your organisation, supply chains, or via management pushing to save resources by using it to draft documents.
As with any change, there are always early adopters – and laggards. As the quality team is naturally risk averse, it can fall to them to ensure that companies adopt AI tools safely, in an ethically sound and compliant way.
This risk aversion can be compounded by the giant leap in our need to understand the technical components that go into these different systems. Many people are unclear about what makes machine learning different from large language models, or even generative AI.
Historically, auditing and checking for compliance has been the backbone of system quality and compliance assurance, but this can be challenging with the ‘Pandora's box’ that is AI.
As organisations increasingly rely on AI systems for decision-making, customer engagement and operational efficiency, ensuring the quality of these systems becomes a pivotal concern for quality professionals.
This article explores the primary hurdles in ensuring the quality of AI in meeting business demands, while making sure quality professionals continue to add value and are not perceived as needless blockers in this leading edge of innovation.
Maintaining transparency
With this objective in mind, we can start at the beginning, where our software teams and developers do their magic with code. I call this magic, because – even with 16 years’ experience as a quality professional – coding still seems to me like alchemists turning lead into gold. I understand it at a surface level, but cannot code myself. However, I still have to ensure that those who can are not just passing off a polished rock in its place.
So, how do we do that when we consider things such as data integrity and bias? Praveen Gujar covers this very topic in ‘Building Trust in AI: Overcoming Bias, Privacy and Transparency Challenges’, which comes back to the principles we all adhere to within our profession – those of careful design and change management.
Another challenge for quality professionals, particularly those who work within the life sciences or under any of the GxPs (Good x Practice), is that old adage: ‘If it isn’t documented, it didn’t happen.’
Many AI systems, particularly those using deep learning, operate as ‘black boxes’, where the decision-making process is opaque, even to developers. This lack of transparency makes it difficult to demonstrate that the required risk assessments and change management have been conducted.
Thankfully, we are not cast adrift, as we do have a life raft in the form of ISO/IEC 42001:2023 Information technology – Artificial intelligence – Management system. This standard offers organisations comprehensive guidance to using AI responsibly and effectively, even as technology evolves rapidly.
It has been designed to cover the various aspects of artificial intelligence and the different applications an organisation may run. The standard provides an integrated approach to managing AI projects, from risk assessment to treating these risks effectively.
As we have seen with other standards, such as ISO 13485:2016 Medical devices – Quality management systems – Requirements for regulatory purposes, regulators widely accept these as best practices, and they give us a common language and point of reference.
Keeping things secure
Depending on your organisation and your role, information security might fall within your remit. As quality professionals, we no longer have to only consider humans when it comes to phishing and cyberattacks.
AI is employed to improve processes worldwide, but ‘bad actors’ also use these tools and are often not as risk averse. They can move incredibly quickly and do not need to follow the transparent change management processes we hold so dear.
I cannot possibly begin to do justice to this topic in this short article, so I would direct readers to Masike Malatji’s work in ‘Artificial Intelligence (AI) Cybersecurity Dimensions: A Comprehensive Framework for Understanding Adversarial and Offensive AI’.
Meeting the challenge
What is the role of quality when it comes to addressing these challenges? Well, this might surprise some, but I see it as the same as it has always been. By that, I mean we need to continue to follow approaches rooted in robust data governance, model transparency, and proactive monitoring, with genuinely scalable quality assurance processes.
As always, the ability to balance efficiency, thoroughness and pragmatism makes the difference between a ‘quality professional’ and a ‘great quality professional’, even when talking about AI. This is never more true than when dealing with the rapid pace of AI innovation, which demands agile quality assurance professionals and practices. Emerging tools such as AI model lifecycle management platforms and advanced simulation environments promise to enhance our efforts.
The bane of my life in quality and auditing has always been the expression ‘But we’ve always done it that way’. At the dawn of this new age, and in the era of Quality 4.0, I hope this saying will become a thing of the past – and we, as quality professionals, cannot fall into the trap of being the ones who now say it.
Instead, we need to do ourselves what we have been helping others do for decades – move through the change curve. We need to change how we think about quality, our place, and what we offer. Organisations must recognise that assuring quality in AI is not a one-time goal, but a continuous journey.
By embedding quality principles into their AI development and deployment workflows, organisations can mitigate risks and build systems that inspire trust and drive sustainable value.
Quality in AI – a case study
My organisation, TQC, has recently partnered with an innovative venture-backed AI startup that is a prime example of an early adopter. With only a handful of employees, a great idea and a developing product, the company has sought to achieve certification to ISO 2700:2022 Information security, cybersecurity and privacy protection – Information security management systems – Requirements to solidify its commitment to protecting sensitive client information.
Our journey began with a comprehensive gap analysis to assess the company’s existing information security management practices against ISO 27001 standards. We’re working closely with the startup’s team to implement robust data governance policies, ensuring compliance with ISO requirements and privacy-specific regulations such as General Data Protection Regulations.
By introducing risk assessment frameworks tailored to the company’s AI workflows, we aim to identify and mitigate vulnerabilities, including those in its model-training pipelines. Additionally, we are developing an incident-response procedure to address potential breaches involving the company’s machine-learning systems.
Through ongoing collaboration, the startup will achieve ISO 27001 certification, and gain a competitive edge by demonstrating its commitment to security and trustworthiness to stakeholders and clients.
This project exemplifies the role of quality professionals in navigating the intersection of innovation and compliance, fostering ethical AI deployment and business growth, while ensuring the processes can scale as the company does.
Summary
With many quality professionals wondering if AI will take our jobs, I see the opposite. This is a new and exciting opportunity for us, as long as we don’t get stuck thinking it’s ‘the way we have always done things’.
If we can help these new tools embed quality and compliance pragmatically, it gives me real hope for the future of quality and compliance. Integrating quality and continual improvement in AI development is not merely an operational necessity, but an ethical imperative. As AI systems increasingly shape our world, ensuring their quality becomes synonymous with safeguarding fairness, accountability and trust.
A commitment to quality and risk management can help organisations navigate this complex terrain, fostering AI solutions that are efficient and resilient. These areas of business quality assurance, information security, data integrity, and privacy protection are where we, as professionals, will continue to add value to any organisation considering making use of these breakthroughs.
Join the CQI's Digital Transformation special interest group
The newly formed Digital Transformation SIG aims to advance the understanding and development of practices of quality management in the digital age.
Quality World
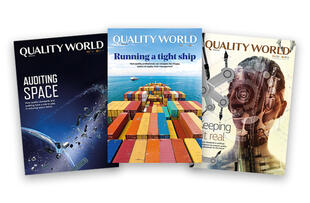
Get the latest news, interviews and features on quality in our industry leading magazine.
Become an event partner for Quality Live 2025
Raise your organisation's profile with our audience of international quality and auditing professionals